Chapter 11 Real-world search
It was the use of certain technologies that helped spur psychologists to study capacity limits, including the problem of fighter pilots having to deal with both flying and monitoring things in the cockpit at the same time. In the aftermath of the September 11 attacks on the U.S., monitoring another aspect of airplanes became prominent: what goes on and off of them.
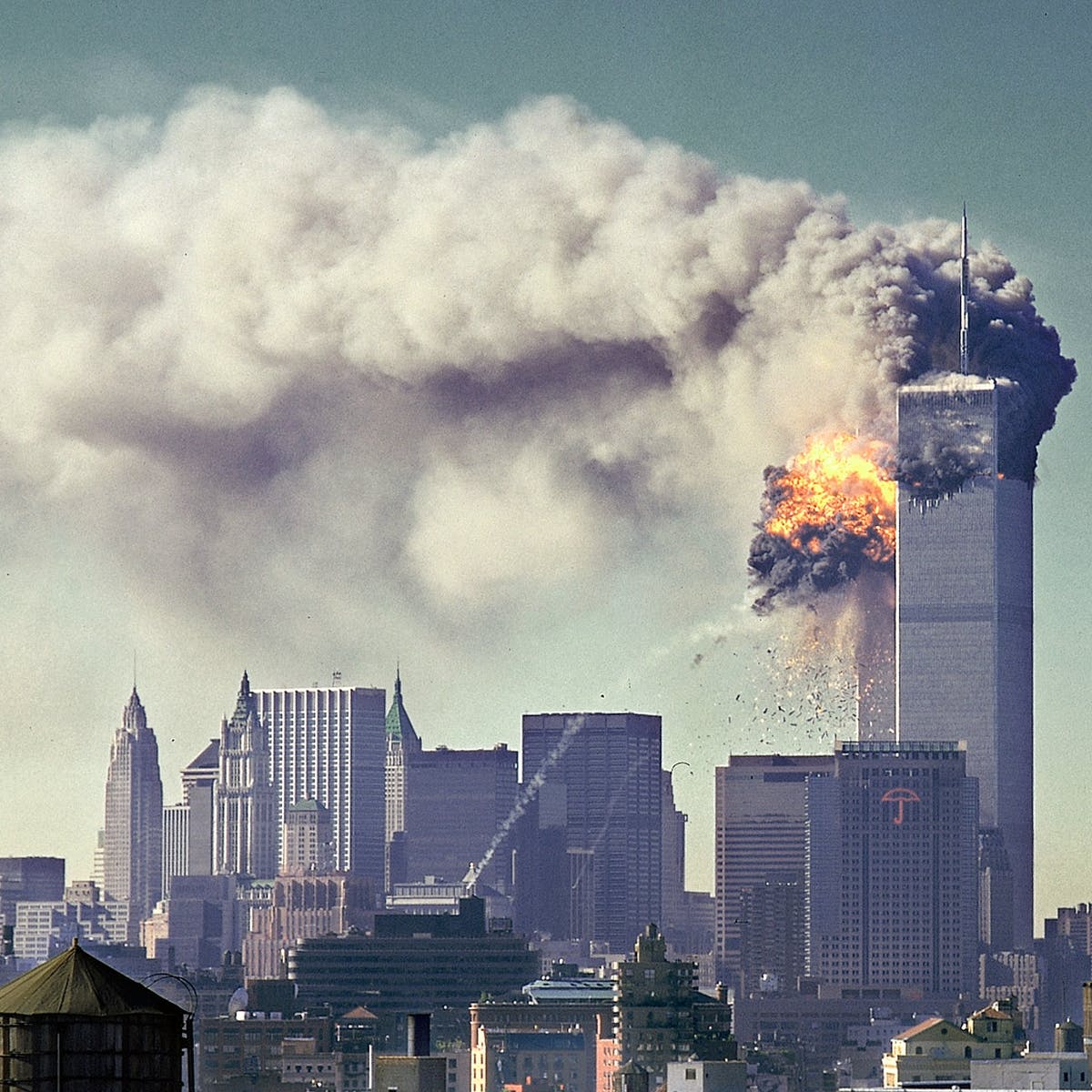
After 2001, tens of thousands of additional workers were hired at airports to search baggage and baggage scanner images for prohibited items.
Which type of response time pattern do you think this kind of search would result in - parallel search or serial search? See chapter 9 for a reminder of what this means.
This kind of search involves additional complications beyond those described in the previous chapter (9), and the baggage inspections result in plenty of mistakes. More than once I’ve accidentally left a prohibited item in my checked baggage or hand baggage, but nevertheless sailed right through.
In 2015, a “sting” operation by the U.S. government reportedly found that baggage screening only caught 5% of bags with (fake) weapons that undercover agents had put in them. Two years later, a similar test found some improvement, to catching about 30% of bags with weapons inserted in them. Still, 30% is clearly a failing mark.
Why did the workers fail to detect such a high proportion of weapons? We don’t know the details, because how the tests were done and the exact pattern of results are secrets. However, from considering the nature of the task more, you should be able to understand the basic issues.
In the searches described in 9, the target and distractors were chosen to be very different from each other. For example, when the target was a red vertical line, the distractors differed greatly on at least one feature, color or orientation. There were never any orange vertical lines, or red slightly-tilted lines. This was to ensure that the search would not be difficult due to the early visual system not being good enough to strongly distinguish between the target and distractor. By using displays that the early visual system can easily distinguish, any findings of poor performance could more confidently be attributed to a bottleneck. This situation was contrasted with searching for a particular word on a page full of text (9.1). When you looked at one part of the page, for most of the rest of the page your vision was too poor to distinguish between different words. There’s a similar problem with looking at an image of a piece of luggage. This means that airport workers need to move their eyes around the image, re-doing the search for each region in the image that their eyes land upon.
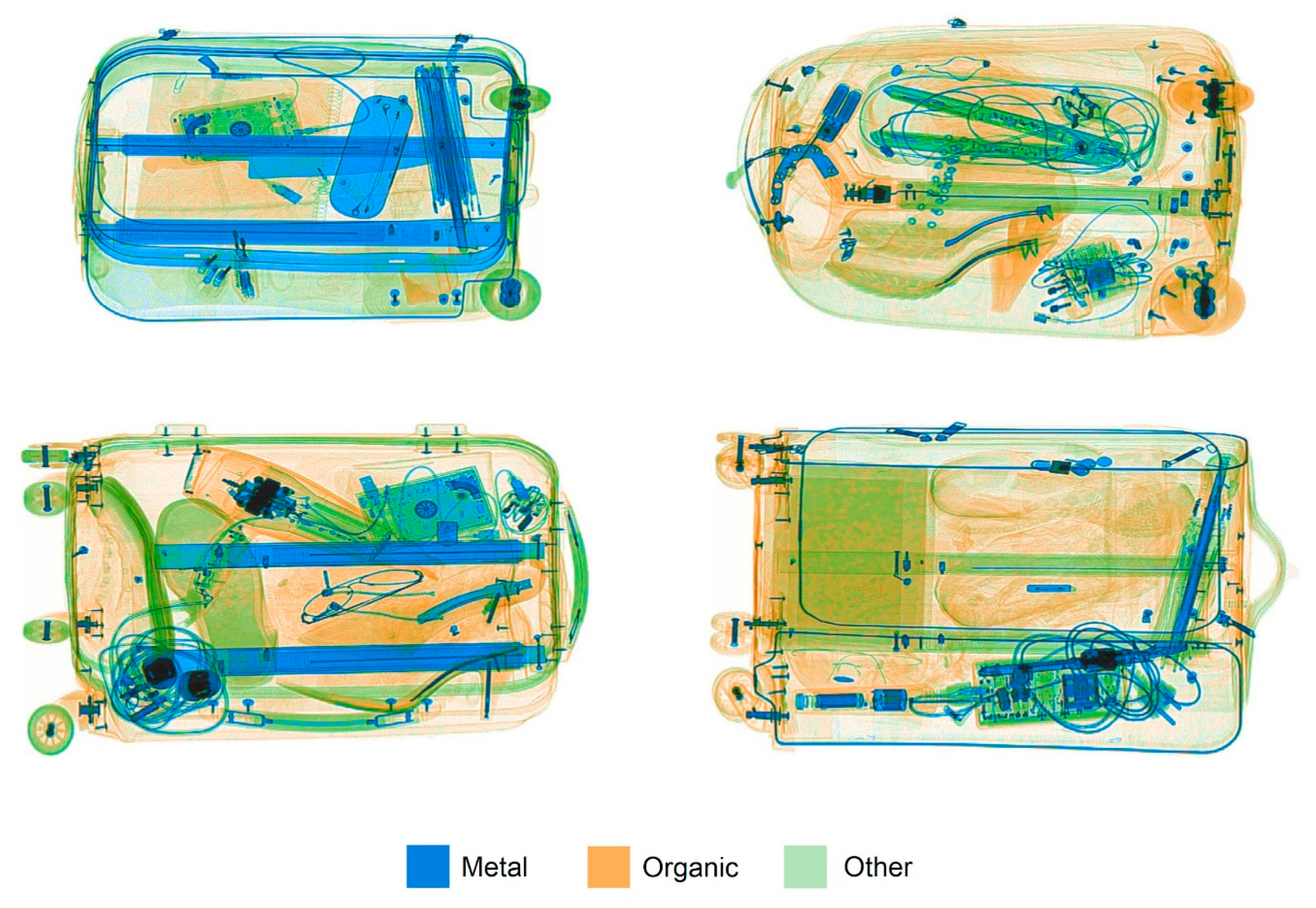
Figure 11.1: X-ray images of bags, two of which contain threats. From Donnelly et al. (2019)
Can you find the prohibited items in the bags above?
Another feature of the laboratory tasks is that participants only had to search for one kind of target at a time, for example for a red vertical line. But airport workers have to keep in mind many targets - scisssors, knives, and lighters as well as guns and explosives. This makes a big difference. Let’s consider what it means for the use of feature selection. Feature selection really helps when the object you are looking for is a unique color, orientation, or simple shape. Unfortunately, prohibited items can be oriented in any direction in a bag and can be any color. The scanner technology does, however, portray metal objects in a single color (blue above), which is helpful because knives and guns are more likely to be metal than made of other materials. So, baggage inspectors can to some extent set their featural attention to blue. Unfortunately, however, even a single contraband category, such as guns, can assume a number of different shapes. The pistol in the bottom right suitcase, for example, has a surprising shape because it is only partly metal and it is pushed against another metal object. So, shape selection ends up not being particularly useful.
Relatedly, even when you look directly at the pistol in the baggage scan, you may not recognise it as being a pistol. This testifies to the need to have expertise to be familiar with all the appearances that a prohibited item can take in a baggage scane.
Finally, laboratory studies have revealed that even in the best of conditions, people are not very good at searching for more than one kind of target at a time. Recall that the capacity limit on full processing of objects means that searches for complex shapes and combinations of features is slow. Something we haven’t talked about is how the brain evaluates whether a fully-processed object representation is a target. To do so, you have to keep what the target is (e.g., “red vertical”) in mind. But baggage inspectors have to keep a whole bunch of targets in mind. In that situation, limitations on memory can impair performance (Wolfe 2012). What sort of memory is used for knowing what the targets are? At first, it may be short-term memory, but after one does the task over and over, it seems to become a form of long-term memory (Wolfe 2020, 13).
In summary, baggage scan searches are difficult for several reasons:
- The targets and distractors can be very similar to each other.
- The targets are not distinguished from the distractors by a single feature.
- The targets do not have a consistent appearance.
- Expertise is necessary to know all the possible ways a target might appear.
- There are several different kinds of target, all of which have to be found.
With multiple factors involved, affecting different mental processes, psychologists’ theories have not advanced enough to accurately predict the performance of people on the baggage scan task. This is true for most real-world tasks. They tend to involve a lot of variation that affects multiple mental processing stages, and our theories are too limited to make good quantitative predictions.
Appropriately engineering a system of humans and machines, then, for baggage inspection or anything else, requires a lot of trial and error. The principles of mental processing inferred from laboratory experiments can guide how a system is designed, but ultimately a lot of tests have to be done to validate those design decisions. For the case of airport baggage inspections, despite a lot of resources devoted to the problem, still the system scores a failing grade. The problem is just too difficult, at least for present scientific understanding and technology.
11.1 Practice and individual differences
Managers of airport security are need to know which people to hire and how to train them. The following, then, are some important questions:
- With practice, do people improve much at detecting banned items?
- How much do people improve?
- With enough training, can anyone be made into an expert and have a similar level of performance?
Ericson, Kravitz, and Mitroff (2017) investigated this by making a game out of airport scanner inspections:
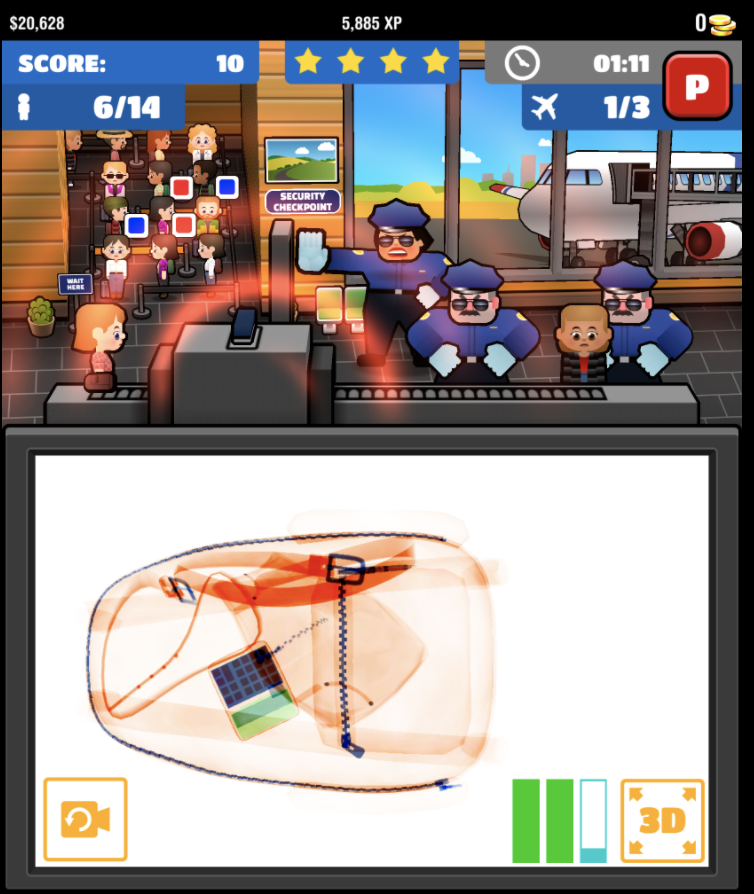
When Ericson, Kravitz, and Mitroff (2017) plotted the data from users of the game, they discovered something important.
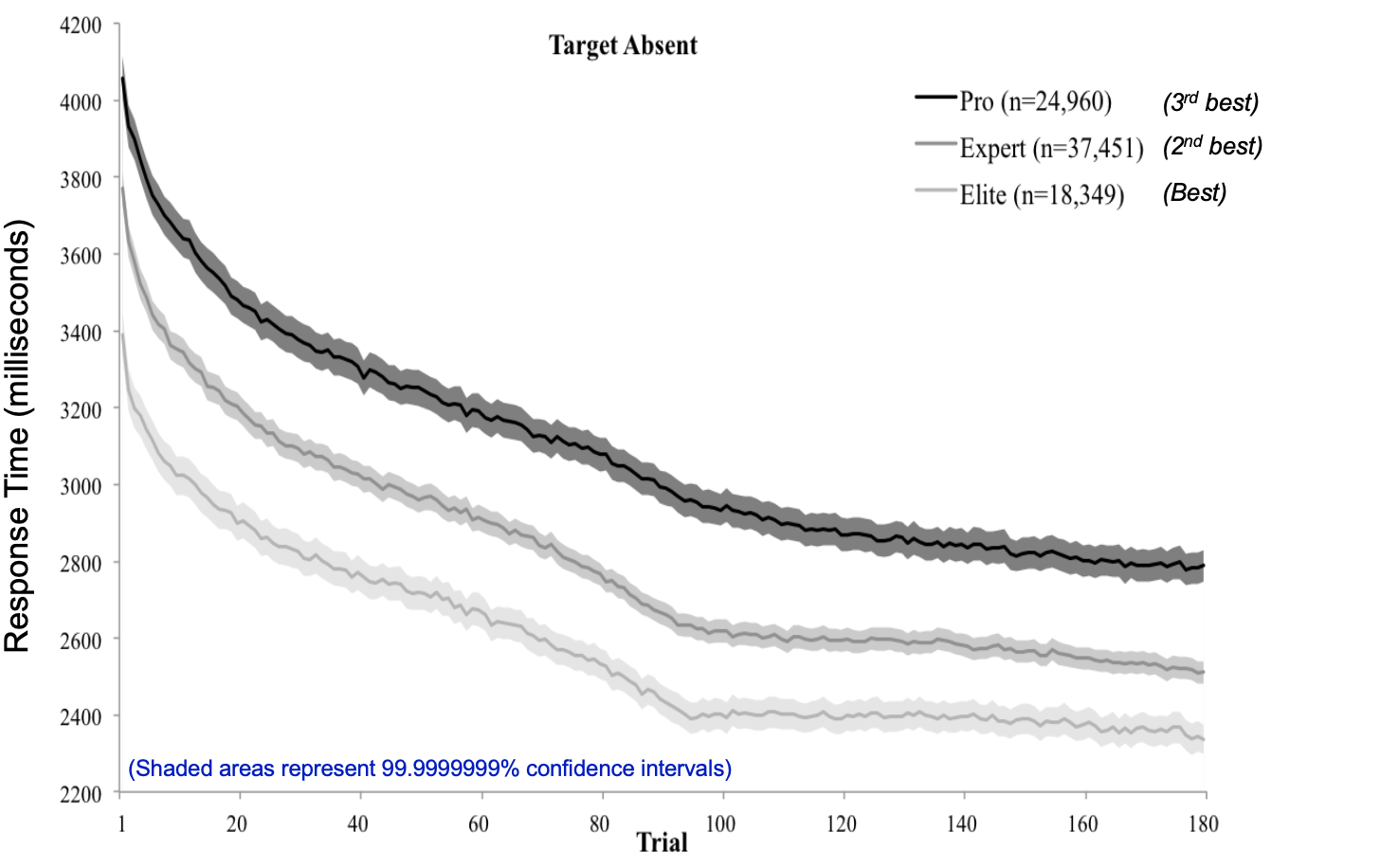
The horizontal axis is trial number, where “trial” means an individual try. The three lines are three different groups of people, those who start out (at trial 1) with relatively slow performance (top line), those who start out a bit faster (middle line) and those who start very fast (bottom line). Each group of players gets better from left to right - the more trials they participate in, the faster their response time.
It’s good that everyone learns with practice. What’s unfortunate, however, is that the curves do not converge - the slow people get much faster but the fast people get even faster, so the people who start out fast maintain their lead. In other words, the individual differences are stable.
From these results, the airport security managers might have some tentative answers to their three questions. What do you suggest for answers to the three questions?